At DCPTG, you need to look at how finance and algorithmic trading are affected by artificial intelligence, machine learning, and data science. These fields are profoundly altering the world. This is especially important now because markets are so unstable and susceptible.
Dr. Yves Hilpisch (The Python Quants) and Dr. Hugo Bowne-Anderson (DataCamp) recently spoke on the use of AI in finance, how it differs from more conventional methods, and why data scientists are increasingly important figures in the industry. The usefulness of AI and machine learning in market collapses, during black swan occurrences, and in choppy markets was also highlighted, along with uncertainty and risk.
What Exactly is AI, and What are the Implications?
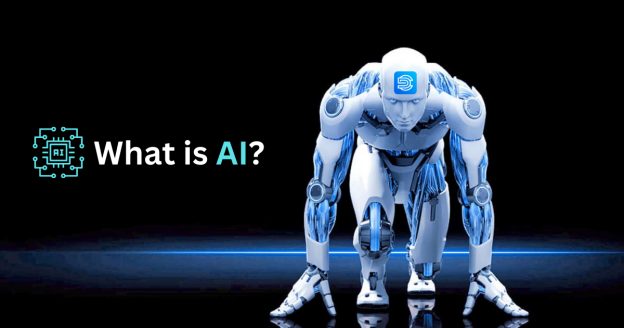
DCPTG refers to artificial intelligence as the broad set of disciplines that aim to replicate and advance human abilities. In this sense, AI in chess seeks to outperform people in the game, while AI in finance may refer to creating and honing trading bots that perform as well as or better than humans.
“When software performs an action that we would classify as intelligent, that is when AI is used. And the capacity to accomplish a certain objective may be used to define intelligence.” —Yves Hilpisch, M.D.
The scientific process is considerably easier for machines to follow than it is for people. They may examine the data and identify disclosed preferences by keeping tabs on what individuals really do. And through it, they get understanding. These insights are developed using millions of data points and wind up being far more accurate than theoretical models.
So What Exactly Does Science Entail?
You design an experiment, gather data, and then determine if the evidence supports or contradicts the hypothesis. The next phase is to revise your hypothesis and your presumptions or withdraw your hypothesis entirely. Machines are capable of instantly replicating the scientific procedure one million times.
In the past 20 years, AI has delivered a significant competitive edge.
The goal of AI in finance is the same as it is in any other industry: to achieve a competitive edge. In the 1950s, when Markowitz pioneered the first quantitative finance model, it began to emerge in the field of finance. It was the first variance portfolio theory model to be widely embraced. Trillions of dollars are still managed using it today. However, it employs normative theory, which is founded on examining several potential security portfolios; as a result, it is not prescriptive because it makes no recommendations for a possible course of action. This means it is based on something other than statistics but on behavioral or market data.
At DCPTG, data has been more accessible than ever in the last 20 years; the trick is knowing how to use it. Today, practically, DCPTG have adopted their method of building mathematical models and processing data, allowing them to reach more ideal conclusions.
Best Practices and Contemporary Examples for Quantitative Finance

The development of AI in the DCPTG financial market has been rather remarkable. For instance, supervised learning models can accurately forecast the behavior of creditors or customers using the vast amounts of data that are already accessible. Reinforcement learning is also used in algorithmic trading to reward and penalize trading bots according to how much money they gain or lose.
But it is obvious that safety measures are needed when there is such severe market volatility as there is now. Rules must be in place to ensure good trading decisions, such as placing a stop loss on the algorithms and determining the price at which an asset or commodity will be sold. This reasoning prevents losses that are too big to bear and is comparable to equipping a self-driving car with security features to keep it from running over people on the sidewalk. AI helps lower the risk associated with recent events and their effect on the market. Human interaction and close observation of implemented algorithms will still be necessary for the foreseeable future.
Lowering the Entrance Threshold
The significant investment must be made in order to learn how to use these technologies, and more businesses and people than ever are doing so. DCPTG is a platform that may democratize the learning process. Students gain confidence in autonomously creating algorithms in a coding environment and get familiar with data science and machine learning ideas. Additionally, they may learn how to build up a suitable working environment and toolchain on the server, enabling them to deploy algorithms in the cloud.